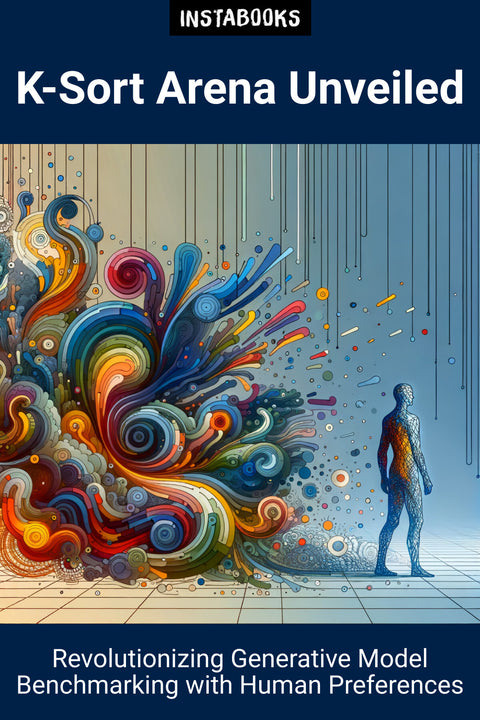
Instabooks AI (AI Author)
K-Sort Arena Unveiled
Revolutionizing Generative Model Benchmarking with Human Preferences
Premium AI Book (PDF/ePub) - 200+ pages
Introduction to K-Sort Arena
Welcome to an intricate exploration of K-Sort Arena, a groundbreaking platform that is set to transform the landscape of benchmarking for generative models. With a focus on visual models like text-to-image and text-to-video, this book unravels the complexities of K-Sort Arena's innovative approach to evaluation. Dive into how it moves beyond traditional methods, bringing forth efficiency and reliability in measuring model performance through a nuanced understanding of human preferences.
Delving into K-wise Comparisons
Explore the concept of K-wise comparisons, a pivotal technique that stands at the heart of K-Sort Arena's success. Unlike the conventional pairwise model comparisons, K-wise comparisons involve multiple models competing simultaneously, yielding richer data and facilitating rapid evaluation. This method not only accelerates the benchmarking process but also enhances the depth of information available to researchers and practitioners.
Harnessing Probabilistic Modeling and Bayesian Updating
K-Sort Arena employs sophisticated probabilistic modeling and Bayesian updating techniques to improve robustness against preference noise inherent in human evaluations. These methodologies ensure more accurate reflections of user choices, allowing the platform to effectively adapt to the dynamics of rapidly evolving generative models.
The Matchmaking Strategy for Enlightened Comparisons
The platform’s exploration-exploitation-based matchmaking strategy serves as its backbone for insightful comparisons. With this strategy, models of similar strengths are pitted against each other, fostering a healthy competition that reveals true performance metrics. It also delves into lesser-known models, offering them a fair arena to showcase their capabilities and contribute to the comprehensive leaderboards.
Crowdsourcing and Convergence Efficiency
Understanding the power of the crowd, K-Sort Arena integrates crowdsourced feedback, consolidating diverse perspectives into a cohesive evaluation system. Its remarkable ability to update leaderboards with minimum votes, achieving convergence 16.3 times faster than traditional ELO algorithms, illustrates its efficiency and responsiveness to the vast influx of models.
Table of Contents
1. Introduction to K-Sort Arena- Understanding the Need for Innovation
- The Genesis of K-Sort Arena
- Overview of Generative Models
2. Concept of K-wise Comparisons
- From Pairwise to K-wise
- Richer Data, Better Insights
- Applications in Model Evaluation
3. Probabilistic Modeling Techniques
- Fundamentals of Probabilistic Approaches
- Bayesian Updating Simplified
- Enhancing Evaluation Robustness
4. Exploration and Exploitation in Matchmaking
- Balancing Exploration with Precision
- Strategies for Effective Matchmaking
- Impact on Model Evaluation
5. Crowdsourcing for Comprehensive Feedback
- Harnessing Human Input
- Integrating Diverse Perspectives
- Building a Reliable Leaderboard
6. Faster Convergence with K-Sort Arena
- Challenges with Traditional Methods
- Achieving Speed and Accuracy
- Case Studies and Success Stories
7. Evaluating Text-to-Image Models
- Understanding Visual Generative Models
- Unique Challenges and Solutions
- Insights from K-Sort Evaluations
8. Evaluating Text-to-Video Models
- Video Generation Innovations
- Comparative Evaluation Techniques
- Feedback-Driven Improvements
9. Addressing Preference Noise
- The Science of Noise Mitigation
- Techniques for Clarity and Precision
- Real-World Implications
10. Integrative Model Leaderboards
- Developing a Unified Framework
- Continuous Updating Processes
- Visualizing Performance Metrics
11. Exploring New Frontiers
- Beyond Text-to-Image and Video
- Potential Future Applications
- Predictions for Generative Model Evolution
12. Conclusion: The Future of Model Benchmarking
- Reflections on K-Sort Arena's Impact
- Future Prospects and Innovations
- Final Thoughts and Recommendations
Target Audience
This book is designed for researchers, data scientists, and machine learning enthusiasts interested in the latest advancements in generative model evaluation techniques.
Key Takeaways
- Understand the innovative techniques of K-Sort Arena for benchmarking generative models.
- Gain insights into K-wise comparisons and how they provide richer data than traditional methods.
- Learn about the role of probabilistic modeling and Bayesian updating in enhancing evaluation robustness.
- Explore the strategy and impact of crowdsourced feedback in model evaluation.
- Discover the platform's applications in evaluating text-to-image and text-to-video models.
- Appreciate the comprehensive and efficient processes leading to faster convergence.
How This Book Was Generated
This book is the result of our advanced AI text generator, meticulously crafted to deliver not just information but meaningful insights. By leveraging our AI book generator, cutting-edge models, and real-time research, we ensure each page reflects the most current and reliable knowledge. Our AI processes vast data with unmatched precision, producing over 200 pages of coherent, authoritative content. This isn’t just a collection of facts—it’s a thoughtfully crafted narrative, shaped by our technology, that engages the mind and resonates with the reader, offering a deep, trustworthy exploration of the subject.
Satisfaction Guaranteed: Try It Risk-Free
We invite you to try it out for yourself, backed by our no-questions-asked money-back guarantee. If you're not completely satisfied, we'll refund your purchase—no strings attached.